The p-value in statistics quantifies the evidence against a null hypothesis. A low p-value suggests data is inconsistent with the null, potentially favoring an alternative hypothesis. Common significance thresholds are 0.05 or 0.01.
Hypothesis testing
When you perform a statistical test, a p-value helps you determine the significance of your results in relation to the null hypothesis.
The null hypothesis (H0) states no relationship exists between the two variables being studied (one variable does not affect the other).
It states the results are due to chance and are not significant in supporting the idea being investigated. Thus, the null hypothesis assumes that whatever you try to prove did not happen.
The alternative hypothesis (Ha or H1) is the one you would believe if the null hypothesis is concluded to be untrue.
The alternative hypothesis states that the independent variable affected the dependent variable, and the results are significant in supporting the theory being investigated (i.e., the results are not due to random chance).
What a p-value tells you
A p-value, or probability value, is a number describing how likely it is that your data would have occurred by random chance (i.e., that the null hypothesis is true).
The level of statistical significance is often expressed as a p-value between 0 and 1.
The smaller the p-value, the less likely the results occurred by random chance, and the stronger the evidence that you should reject the null hypothesis.
Remember, a p-value doesn’t tell you if the null hypothesis is true or false. It just tells you how likely you’d see the data you observed (or more extreme data) if the null hypothesis was true.
It’s a piece of evidence, not a definitive proof.
Example: Test Statistic and p-Value
Suppose you’re conducting a study to determine whether a new drug has an effect on pain relief compared to a placebo.
If the new drug has no impact, your test statistic will be close to the one predicted by the null hypothesis (no difference between the drug and placebo groups), and the resulting p-value will be close to 1. It may not be precisely 1 because real-world variations may exist.
Conversely, if the new drug indeed reduces pain significantly, your test statistic will diverge further from what’s expected under the null hypothesis, and the p-value will decrease.
The p-value will never reach zero because there’s always a slim possibility, though highly improbable, that the observed results occurred by random chance.
How to Choose an Alpha Level (Significance Threshold)
Choosing an alpha level (α) depends on your research goals, context, and potential impacts:
-
Standard Alpha (α = .05): This is the most frequently used level. It means you accept a 5% chance (1 in 20) of mistakenly finding an effect when there isn’t one (known as a Type I error). Ideal for exploratory or general research.
-
Stricter Alpha (α = .01 or .001): Used for high-stakes research, like clinical trials, mental health interventions, or policy decisions, where mistakes have serious consequences. A lower alpha reduces the chance of false positives (finding something significant that isn’t actually there).
-
Study Size and Reliability: Larger studies (typically 200 or more participants) often let you safely use a stricter alpha (e.g., α = .01), because larger samples provide more reliable results.
-
Practical Implications: If making a wrong decision could have major consequences (like health or safety risks), choose a lower alpha to minimize mistakes.
Clearly state and justify your chosen alpha level to ensure transparency and improve the trustworthiness of your findings.
P-value interpretation
The significance level (alpha) is a chosen threshold (often 0.05), while the p-value is the probability calculated from your data.
P-Value | Interpretation | Action |
---|---|---|
≤ 0.01 | Very strong evidence against null hypothesis | Reject null hypothesis |
≤ 0.05 | Strong evidence against null hypothesis | Reject null hypothesis |
> 0.05 | Weak/no evidence against null hypothesis | Fail to reject null |
A p-value less than or equal to your significance level (typically ≤ 0.05) is statistically significant.
A p-value less than or equal to a predetermined significance level (often 0.05 or 0.01) indicates a statistically significant result, meaning the observed data provide strong evidence against the null hypothesis.
This suggests the effect under study likely represents a real relationship rather than just random chance.
For instance, if you set α = 0.05, you would reject the null hypothesis if your p-value ≤ 0.05.
It indicates strong evidence against the null hypothesis, as there is less than a 5% probability the null is correct (and the results are random).
Therefore, we reject the null hypothesis and accept the alternative hypothesis.
Example: Statistical Significance
Upon analyzing the pain relief effects of the new drug compared to the placebo, the computed p-value is less than 0.01, which falls well below the predetermined alpha value of 0.05.
Consequently, you conclude that there is a statistically significant difference in pain relief between the new drug and the placebo.
What does a p-value of 0.001 mean?
A p-value of 0.001 is highly statistically significant beyond the commonly used 0.05 threshold. It indicates strong evidence of a real effect or difference, rather than just random variation.
Specifically, a p-value of 0.001 means there is only a 0.1% chance of obtaining a result at least as extreme as the one observed, assuming the null hypothesis is correct.
Such a small p-value provides strong evidence against the null hypothesis, leading to rejecting the null in favor of the alternative hypothesis.
A p-value more than the significance level (typically p > 0.05) is not statistically significant and indicates strong evidence for the null hypothesis.
This means we retain the null hypothesis and reject the alternative hypothesis. You should note that you cannot accept the null hypothesis; we can only reject it or fail to reject it.
Note: when the p-value is above your threshold of significance, it does not mean that there is a 95% probability that the alternative hypothesis is true.
One-Tailed Test
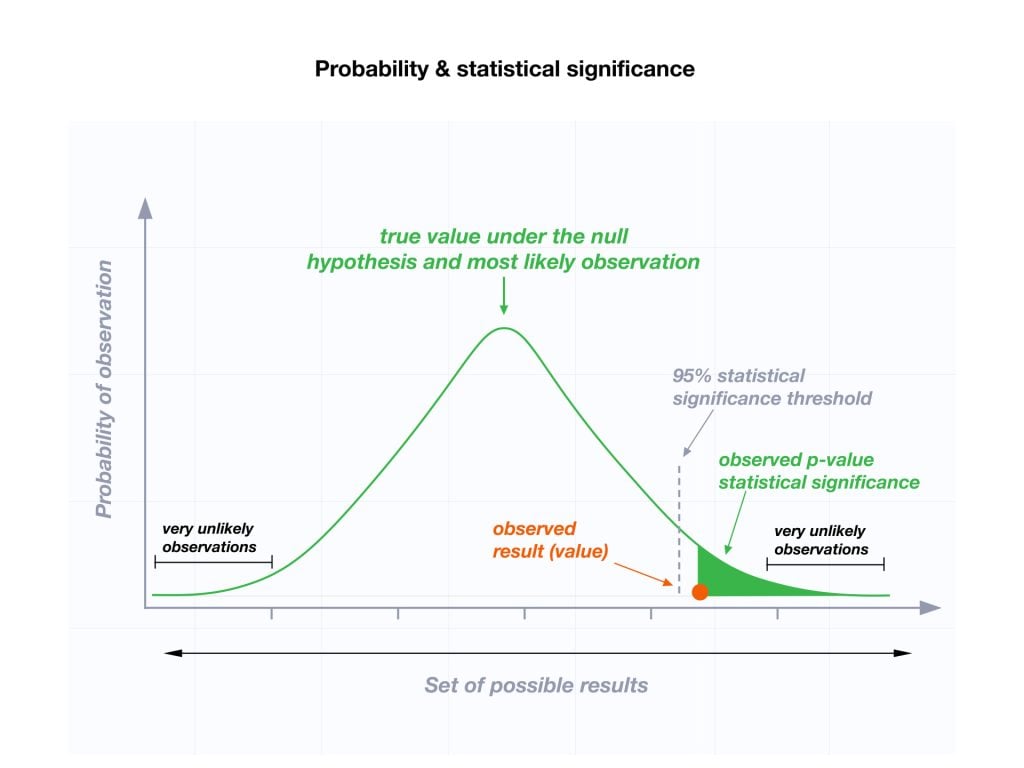
Two-Tailed Test
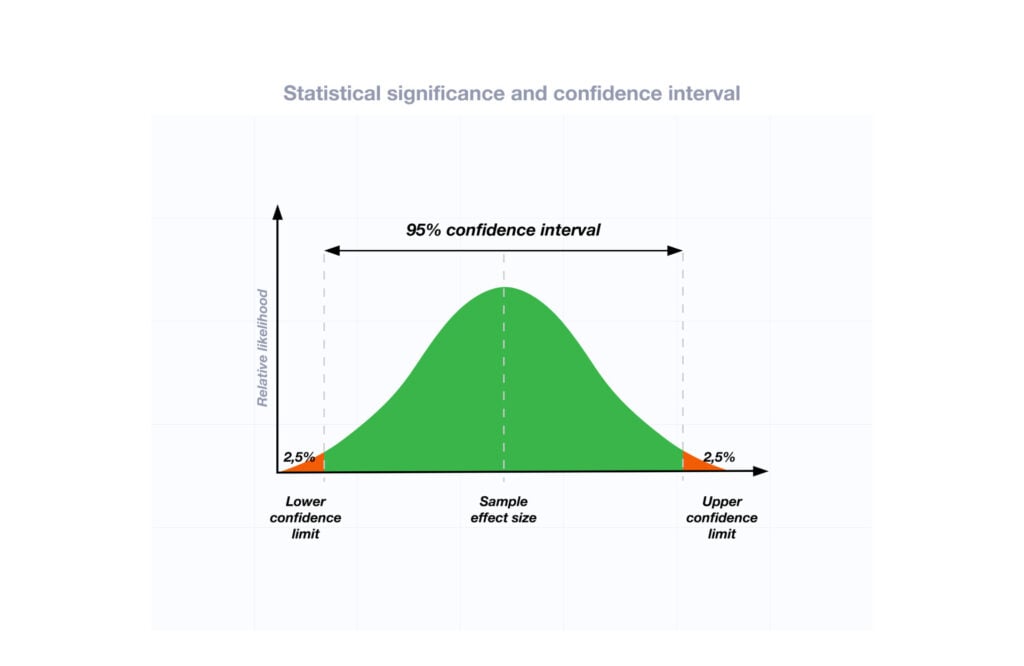
Type I and Type II Errors
-
Type I Error (False Positive): This occurs when you incorrectly find an effect or difference that doesn’t really exist. For instance, concluding a medication improves symptoms when it actually does not. With an alpha (α) level of .05, there’s a 5% chance of making this error.
-
Type II Error (False Negative): This happens when you fail to detect an effect that genuinely exists. For example, concluding a medication doesn’t help when it truly does. You can reduce this error by increasing your sample size or improving your research design.
How do you calculate the p-value?
Most statistical software packages like R, SPSS, and others automatically calculate your p-value. This is the easiest and most common way.
Online resources and tables are available to estimate the p-value based on your test statistic and degrees of freedom.
These tables help you understand how often you would expect to see your test statistic under the null hypothesis.
Understanding the Statistical Test:
Different statistical tests are designed to answer specific research questions or hypotheses. Each test has its own underlying assumptions and characteristics.
Each statistical test serves specific research questions and has particular assumptions:
-
T-test: Used to compare the means of two groups (e.g., testing if one medication is more effective than another).
-
ANOVA (Analysis of Variance). Comparing scores across three groups (e.g. therapy, medication, and combined treatment).
-
Chi-squared test: Suitable for categorical data (e.g., evaluating the relationship between smoking status and lung disease).
-
Correlation test: Measures the strength and direction of relationships between two continuous variables (e.g., height and weight).
Example: Choosing a Statistical Test
The more variables you include, the more careful you must be interpreting your p-values.
More variables can affect your test statistic, potentially leading to misleading significance.
If you’re comparing the effectiveness of just two different drugs in pain relief, a two-sample t-test is a suitable choice for comparing these two groups. However, when you’re examining the impact of three or more drugs, it’s more appropriate to employ an Analysis of Variance (ANOVA).
Utilizing multiple pairwise comparisons in such cases can lead to artificially low p-values and an overestimation of the significance of differences between the drug groups.
How to report
A statistically significant result cannot prove that a research hypothesis is correct (which implies 100% certainty).
Instead, we may state our results “provide support for” or “give evidence for” our research hypothesis (as there is still a slight probability that the results occurred by chance and the null hypothesis was correct – e.g., less than 5%).
Example: Reporting the results
In our comparison of the pain relief effects of the new drug and the placebo, we observed that participants in the drug group experienced a significant reduction in pain (M = 3.5; SD = 0.8) compared to those in the placebo group (M = 5.2; SD = 0.7), resulting in an average difference of 1.7 points on the pain scale (t(98) = -9.36; p < 0.001).
APA Style
The 6th edition of the APA style manual (American Psychological Association, 2010) states the following on the topic of reporting p-values:
“When reporting p values, report exact p values (e.g., p = .031) to two or three decimal places. However, report p values less than .001 as p < .001.
The tradition of reporting p values in the form p < .10, p < .05, p < .01, and so forth, was appropriate in a time when only limited tables of critical values were available.” (p. 114)
Note:
- Do not use 0 before the decimal point for the statistical value p as it cannot equal 1. In other words, write p = .001 instead of p = 0.001.
- Please pay attention to issues of italics (p is always italicized) and spacing (either side of the = sign).
- p = .000 (as outputted by some statistical packages such as SPSS) is impossible and should be written as p < .001.
- The opposite of significant is “nonsignificant,” not “insignificant.”
Why is the p-value not enough?
A lower p-value is sometimes interpreted as meaning there is a stronger relationship between two variables.
However, a p-value simply tells you how unlikely your data is if the null hypothesis is true (less than 5%), it doesn’t reveal the size or practical importance of the effect.
To fully understand your findings, researchers should also report the effect size and confidence intervals.
Relationship Between Effect Size, Confidence Intervals, and P-Values
Effect size, confidence intervals, and p-values each contribute uniquely and complementarily to statistical interpretation:
-
Effect Size: Shows how large or meaningful the observed effect is in practical terms. For example, in mental health research, a therapy might significantly reduce anxiety scores, but if the decrease is minimal (e.g., 1-2 points on a 50-point scale), the practical significance could be limited.
-
Confidence Intervals (CIs): Provide a range where the true effect likely lies, usually set at 95%. Narrower intervals imply greater precision in estimating the effect. For example, an anxiety reduction study reporting a confidence interval of 5 to 10 points clearly suggests a reliable treatment effect.
-
P-Values: Show how likely the observed data would be if the null hypothesis were true, assessing statistical significance.
For example, a very small p-value (such as 0.001) in a depression intervention trial suggests strong statistical evidence that the intervention had some effect, but without effect size, it doesn’t indicate if the change is clinically meaningful.
Combining These Concepts:
- Small p-value + Large effect size + Narrow CI: Strong evidence that the effect is real and practically significant.
- Small p-value + Small effect size: Indicates statistical significance, but the practical impact may be limited.
- Significant p-value + Wide CI: Suggests uncertainty about the exact size of the effect, indicating caution in interpreting results.
Reporting effect sizes, confidence intervals, and p-values together ensures thorough, transparent, and meaningful interpretation of your research findings.
FAQs
When do you reject the null hypothesis?
In statistical hypothesis testing, you reject the null hypothesis when the p-value is less than or equal to the significance level (α) you set before conducting your test.
The significance level is the probability of rejecting the null hypothesis when it is true. Commonly used significance levels are 0.01, 0.05, and 0.10.
Remember, rejecting the null hypothesis doesn’t prove the alternative hypothesis; it just suggests that the alternative hypothesis may be plausible given the observed data.
The p -value is conditional upon the null hypothesis being true but is unrelated to the truth or falsity of the alternative hypothesis.
What does p-value of 0.05 mean?
If your p-value is less than or equal to 0.05 (the significance level), you would conclude that your result is statistically significant.
This means the evidence is strong enough to reject the null hypothesis in favor of the alternative hypothesis.
Are all p-values below 0.05 considered statistically significant?
No, not all p-values below 0.05 are considered statistically significant. The threshold of 0.05 is commonly used, but it’s just a convention.
Statistical significance depends on factors like the study design, sample size, and the magnitude of the observed effect.
A p-value below 0.05 means there is evidence against the null hypothesis, suggesting a real effect. However, it’s essential to consider the context and other factors when interpreting results.
Researchers also look at effect size and confidence intervals to determine the practical significance and reliability of findings.
How does sample size affect the interpretation of p-values?
Sample size can impact the interpretation of p-values. A larger sample size provides more reliable and precise estimates of the population, leading to narrower confidence intervals.
With a larger sample, even small differences between groups or effects can become statistically significant, yielding lower p-values. In contrast, smaller sample sizes may not have enough statistical power to detect smaller effects, resulting in higher p-values.
Therefore, a larger sample size increases the chances of finding statistically significant results when there is a genuine effect, making the findings more trustworthy and robust.
Can a non-significant p-value indicate that there is no effect or difference in the data?
No, a non-significant p-value does not necessarily indicate that there is no effect or difference in the data. It means that the observed data do not provide strong enough evidence to reject the null hypothesis.
There could still be a real effect or difference, but it might be smaller or more variable than the study was able to detect.
Other factors like sample size, study design, and measurement precision can influence the p-value. It’s important to consider the entire body of evidence and not rely solely on p-values when interpreting research findings.
Can P values be exactly zero?
While a p-value can be extremely small, it cannot technically be absolute zero.
When a p-value is reported as p = 0.000, the actual p-value is too small for the software to display. This is often interpreted as strong evidence against the null hypothesis. For p values less than 0.001, report as p < .001
My experiment yielded a p-value of 0.06. Should I discard my hypothesis entirely? How should I interpret this result accurately?
A p-value of 0.06 means there’s only a 6% chance your results would occur if there’s truly no effect—still relatively low.
Being close to significance might suggest there could be an effect, but your evidence isn’t strong enough yet.
You might have encountered a Type II error (failing to detect a real effect).
If your sample size is small or your study has low statistical power (below 80%), you may have missed detecting an actual effect.
Look at the effect size: is it meaningful enough to matter in real-world scenarios?
A practically significant effect could still be important, even without statistical significance.
If your confidence interval is narrow and mostly suggests a meaningful effect, this increases confidence that the result may still have practical value.
A wide interval suggests greater uncertainty, making conclusions less reliable.
Assess if there were methodological limitations or biases (like measurement error or uncontrolled variables) that may have weakened your results.
Check if other research supports similar findings or trends. If consistent with existing literature, your result could still be meaningful.
Further Information
- P Value Calculator From T Score
- P-Value Calculator For Chi-Square
- P-values and significance tests (Kahn Academy)
- Hypothesis testing and p-values (Kahn Academy)
- Wasserstein, R. L., Schirm, A. L., & Lazar, N. A. (2019). Moving to a world beyond “ p “< 0.05”.
- Criticism of using the “ p “< 0.05”.
- Publication manual of the American Psychological Association
- Statistics for Psychology Book Download
Sources:
Bland, J. M., & Altman, D. G. (1994). One and two sided tests of significance: Authors’ reply. BMJ: British Medical Journal, 309(6958), 874.
Goodman, S. N., & Royall, R. (1988). Evidence and scientific research. American Journal of Public Health, 78(12), 1568-1574.
Goodman, S. (2008, July). A dirty dozen: twelve p-value misconceptions. In Seminars in hematology (Vol. 45, No. 3, pp. 135-140). WB Saunders.
Lang, J. M., Rothman, K. J., & Cann, C. I. (1998). That confounded P-value. Epidemiology (Cambridge, Mass.), 9(1), 7-8.